Table of Contents
In the ever-evolving landscape of artificial intelligence, a remarkable breakthrough has emerged – Cheetor, a revolutionary Transformer-based (MLLM). This cutting-edge innovation has taken the AI community by storm, demonstrating unprecedented proficiency in handling an extensive array of interleaved vision and language instructions, achieving groundbreaking results in zero-shot learning. In this comprehensive article, we delve deep into the intricacies of Cheetor, exploring its architecture, capabilities, and the profound implications it holds for the future of AI.
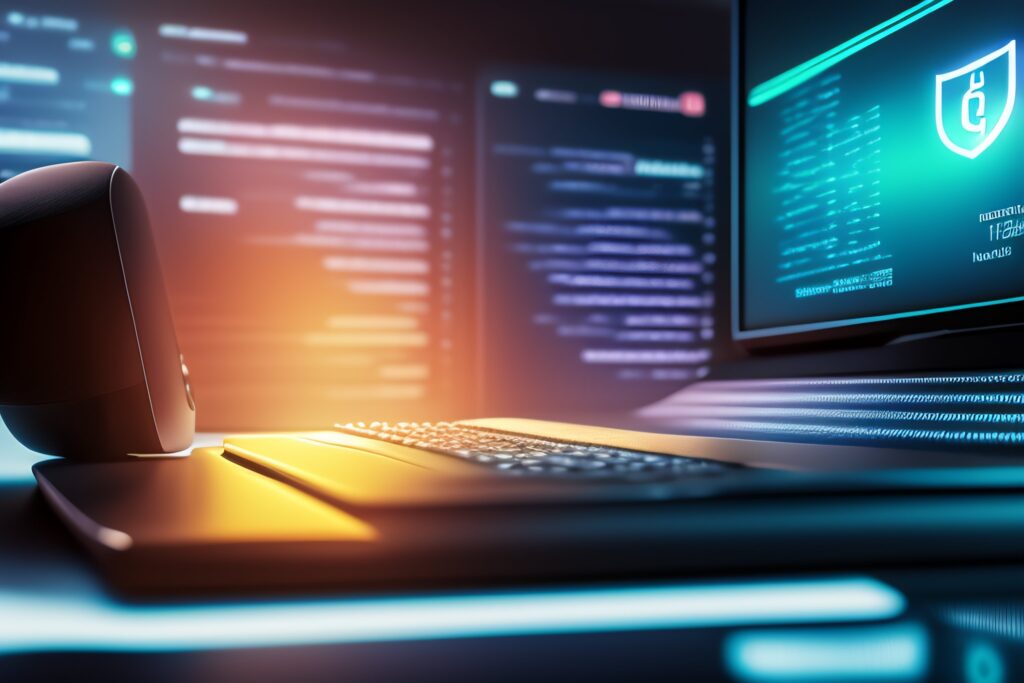
Understanding Cheetor’s Architecture
Cheetor’s architecture is a marvel in itself, comprising multiple layers of intricate design meticulously crafted to seamlessly integrate vision and language processing. At its core lies the Transformer architecture, which has proven to be a foundational pillar in various AI advancements. However, what sets Cheetor apart is its enhanced adaptability to process multimodal inputs. Through a fusion of vision and language encoders, Cheetor establishes cross-modal connections that facilitate a holistic comprehension of the input data.
The Power of Multimodal Comprehension
One of the standout features of Cheetor is its remarkable ability to effectively handle interleaved vision and language instructions. Traditional models often struggled to bridge the gap between these two modalities, resulting in suboptimal performance. Cheetor, however, excels in interpreting intricate contexts where vision and language intertwine. This proficiency opens doors to a myriad of real-world applications, from advanced human-machine interaction to content generation that seamlessly blends visual and textual elements.
Achieving State-of-the-Art Zero-Shot Learning
Zero-shot learning has long been a tantalizing goal in the AI realm, and Cheetor brings us one step closer to realizing it. By leveraging its robust multimodal foundation, Cheetor demonstrates unparalleled prowess in zero-shot learning scenarios. This means that the model can generalize its understanding to tasks it has never been explicitly trained on, showcasing a level of adaptability and comprehension that was previously deemed unattainable.
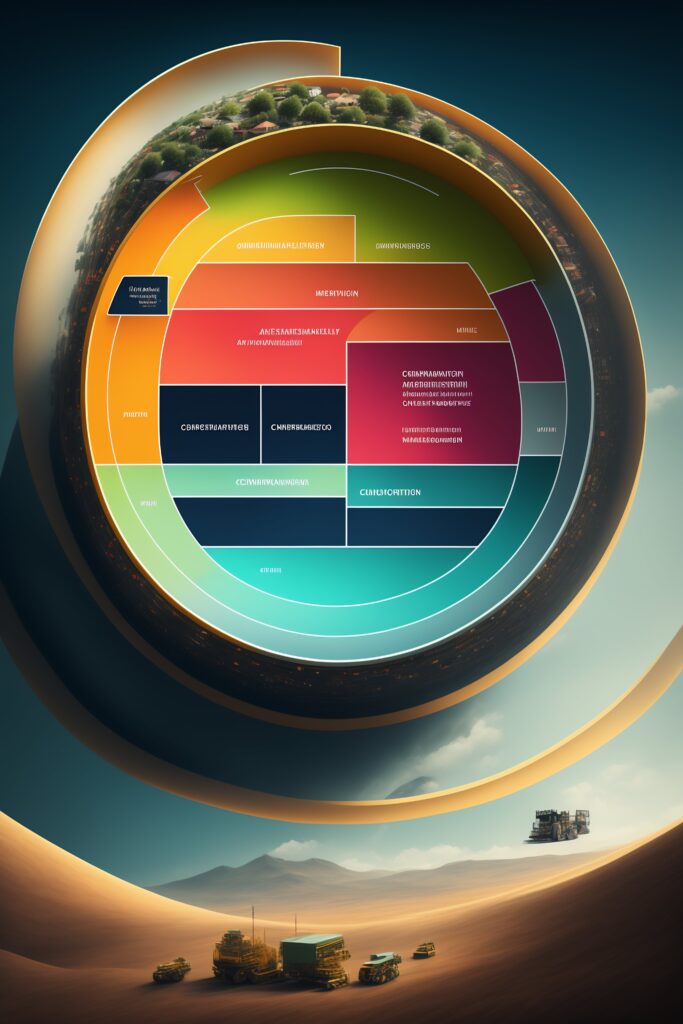
Implications for Various Industries
The implications of Cheetor’s capabilities reverberate across a plethora of industries. In healthcare, this technology could facilitate more accurate diagnosis by comprehending medical imagery and patient descriptions simultaneously. In e-commerce, Cheetor could enhance the user experience by generating product descriptions that seamlessly integrate images and text. Moreover, the entertainment industry could benefit from immersive storytelling experiences that combine visual and narrative elements in unprecedented ways.
The Future of AI: Cheetor’s Legacy
Cheetor’s emergence marks a pivotal moment in the trajectory of AI development. As we stand on the cusp of a new era, where multimodal understanding is paramount, Cheetor’s architecture and capabilities pave the way for groundbreaking applications. The fusion of vision and language processing, along with its state-of-the-art zero-shot learning, promises a future where AI systems seamlessly comprehend and interact with the world in a manner that was once purely science fiction.
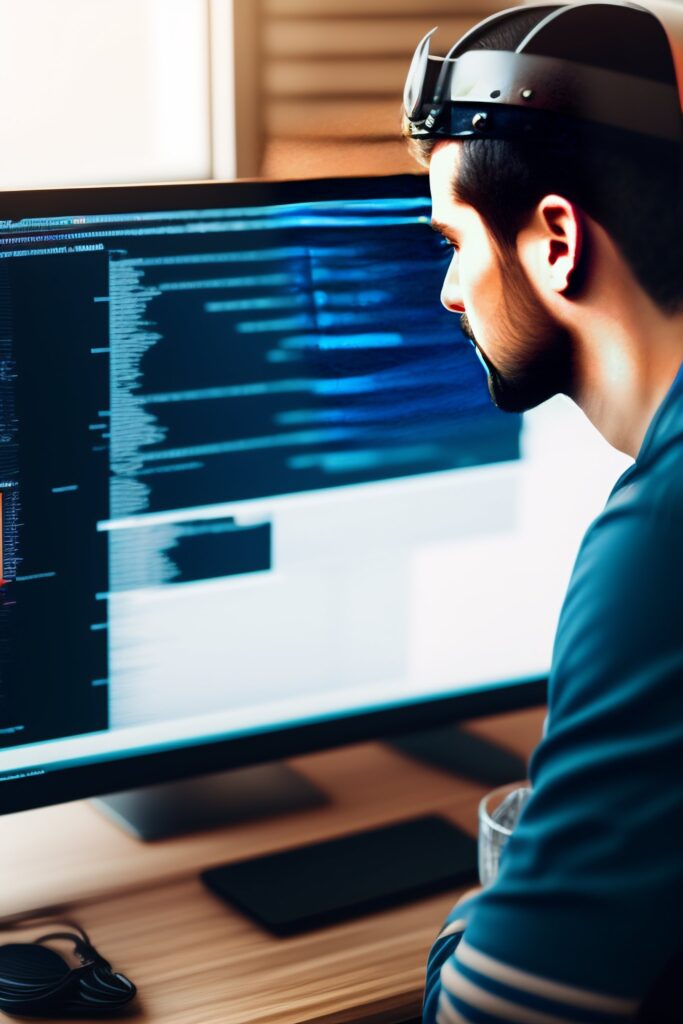
FAQ’s
Q: What is Cheetor?
A: Cheetor is a revolutionary Transformer-based Multimodal Large Language Model (MLLM) designed to seamlessly process both vision and language inputs.
Q: How does Cheetor differ from traditional AI models?
A: Unlike traditional models, Cheetor excels in handling interleaved vision and language instructions, enabling holistic comprehension of complex data.
Q: What is the core architecture of Cheetor?
A: Cheetor’s core architecture is based on the Transformer model, which is enhanced to accommodate both vision and language processing.
Q: What is the significance of multimodal comprehension?
A: Multimodal comprehension allows Cheetor to interpret inputs that blend visual and textual data, enabling it to understand complex contexts more effectively.
Q: How does Cheetor achieve zero-shot learning?
A: Cheetor’s strong foundation in multimodal understanding empowers it to generalize knowledge and excel in tasks it hasn’t been explicitly trained for.
Q: What are the practical applications of Cheetor?
A: Cheetor’s applications range from healthcare diagnosis using medical imagery and patient descriptions to enhancing e-commerce user experiences with blended content.
Q: Can Cheetor generate content?
A: Yes, Cheetor’s capabilities extend to content generation, seamlessly integrating visual and textual elements.
Q: Is Cheetor suitable for the entertainment industry?
A: Absolutely, Cheetor could revolutionize storytelling by crafting immersive narratives that combine visual and narrative aspects.
Q: How is Cheetor’s architecture visualized?
A: Cheetor’s architecture can be visualized through a simplified Mermaid diagram showcasing input data, language and vision encoders, and the Transformer layers.
Q: What industries could benefit from Cheetor’s capabilities?
A: Industries like healthcare, e-commerce, education, and entertainment could harness Cheetor’s potential to enhance various aspects of their operations.
Q: Can Cheetor understand and process real-time data?
A: Yes, Cheetor’s architecture enables it to handle real-time data processing, making it suitable for applications that require rapid responses.
Q: How does Cheetor handle ambiguous or complex instructions?
A: Cheetor’s multimodal comprehension aids in disambiguating complex instructions by combining visual and linguistic cues.
Q: Is Cheetor adaptable to new tasks?
A: Yes, Cheetor’s zero-shot learning capability allows it to adapt to new tasks without specific training, showcasing its versatility.
Q: What potential challenges does Cheetor face?
A: While Cheetor’s capabilities are remarkable, challenges like bias mitigation and ethical considerations must be addressed to ensure responsible usage.
Q: How does Cheetor’s efficiency compare to other models?
A: Cheetor’s efficiency varies based on the complexity of tasks, but its unique architecture positions it as a strong contender in the AI landscape.
Q: Can Cheetor understand multiple languages?
A: Yes, Cheetor’s language understanding extends to multiple languages, enhancing its global applicability.
Q: What kind of training data does Cheetor require?
A: Cheetor’s training data encompasses multimodal inputs that include both visual and language components, enabling it to learn effectively.
Q: Is Cheetor’s architecture open for research and development?
A: While specifics may vary, many aspects of Cheetor’s architecture are likely to be shared with the research and development community.
Q: How does Cheetor’s performance scale with increased data volume?
A: Cheetor’s performance generally benefits from increased data volume, as it enhances its ability to generalize and comprehend diverse contexts.
Q: What does the future hold for Cheetor?
A: The future of Cheetor is promising, with potential advancements in areas like advanced human-machine interaction, AI-driven creativity, and more.

Conclusion
In conclusion, Cheetor’s transformative potential is undeniable. With its ability to handle interleaved vision and language instructions, achieve zero-shot learning, and revolutionize various industries, it stands as a testament to the power of innovation in the AI landscape. As we look ahead, the legacy of Cheetor will undoubtedly shape the evolution of AI, opening doors to possibilities we’ve only begun to explore.
4 thoughts on “Unveiling Cheetor: The Ultimate Transformer-Based Multimodal Large Language Model (MLLM)”